- April 2024
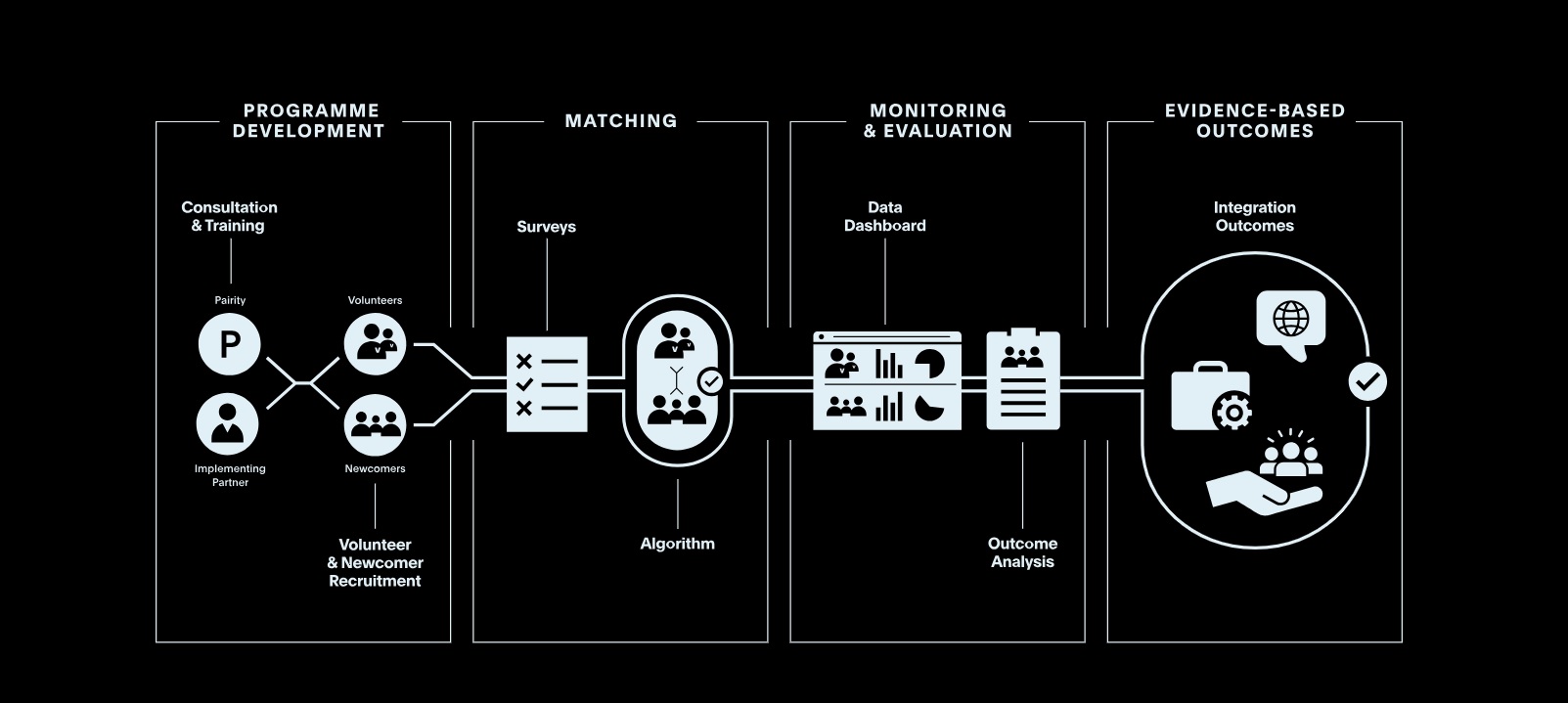
This article discusses real-world projects using algorithms to match resettled refugees with sponsors and services. The authors argue that when done right, algorithms can support larger-scale and better-informed resettlement.
Techno-scepticism and techno-optimism
Research and advocacy around data and tech to manage international mobility can be divided into techno-sceptical and techno-optimistic camps. While the dichotomy is admittedly a broad heuristic, these parallel research tracks draw attention to the inherently dual-use function of any technology.
Techno-sceptical research and advocacy raise well-founded concerns around uses like biometrics and surveillance in border controls, automated visa decisions, or artificial intelligence (AI) for predicting asylum or displacement trends. It is largely informed by a commitment to migrants’ rights to move and seek protection. For example, sceptical research calls attention to the ethical implications of data-gathering for monitoring migrants, the absence of recourse to appeal for automated decisions, and the threat of hard-coding group-based biases. In short, it focuses on the use of technology to curtail ‘unwanted’ migration, rather than facilitating international mobility.
One of the major unstated premises in techno-sceptical literature is the assumption that existing decision-making systems are somehow fairer and less biased than using data or tech. However, human decisions are at least equally (though probably more) prone to error, bias and subjective value judgments. When applied to refugee resettlement, for example, bureaucracies and civil society organisations rarely, if ever, keep verifiable records of why refugees are placed in specific locations, or the rationale behind decisions to match individuals or households with community sponsors or to specific locations.
On the techno-optimist side, experimental work using historical data has shown that algorithms can significantly improve integration outcomes for newly resettled refugees, particularly around labour market performance. Research in the UK, Switzerland and the United States indicates that using algorithms to match refugees with destinations can significantly enhance employment outcomes. The drawback of this approach is that it can often flatten peoples’ life courses to merely economic indicators, rely on unverified assumptions about refugees’ priorities and aspirations, and raise ethical concerns around genuinely informed consent.
These parallel veins of research take place amid growing concern about the role of algorithms and AI in social and political fields. Countries and supranational organisations, in particular the European Union (EU), are working to catch up with the rapid pace of technological change by regulating AI and algorithms. This extends to their deployment in immigration and asylum policy, which the European Commission designated as a ‘high risk’ domain due to the vulnerability of affected populations and concerns about fundamental rights
A middle path: algorithm-supported interventions
While the two camps of advocacy and research rarely engage in dialogue, they offer complementary insights into how applying tech to international mobility is far from zero-sum. Our experience matching refugees with sponsors and services in North America and Europe has shown that simply using the term ‘algorithm’ can lead to immediate ethical concerns, but also that algorithms are often conflated with AI – suggesting that algorithms use big data or train on inherently biased sources. For example, in the Re:Match programme, our algorithms suggest optimal matches for relocating displaced Ukrainians from Poland to Germany – which are then vetted and approved by programme staff. Journalists who interviewed us about the project started with questions around bias and handing over decisions to machines. The line of questioning is both valid and welcome, but also typical of assumptions about how algorithms work in practice.
Algorithms can be written to facilitate mobility and improve outcomes, just as they can be trained to reject visas for nationalities deemed a ‘risk’ for asylum claims. Most often, applied algorithms are simply computational tools for addressing the complex problem of sorting through large amounts of data to optimise scarce resource allocation – like spots in community sponsorship programs, affordable housing, or services for refugees with particular needs. Once they are shown how ethically-informed algorithms can be used to facilitate rather than control migration, civil society actors understand the value of such algorithms in supporting resettlement work.
At the most fundamental level, algorithmic matching can help scale resettlement – as we explored in a Migration Policy Institute policy paper. First, it frees up human resources to directly support refugees in the resettlement process and to focus on advocacy around upholding and improving international protection laws and norms. Second, it can improve resettlement by using objective rules to ensure a good fit between refugees and their destinations, promoting self-reliance as early as possible.
Matching refugees with destinations or community sponsors entails collecting, storing, and analysing large amounts of data. Running these procedures ‘by hand’ is incredibly labour-intensive, quickly runs into barriers to scaling and introduces inherent bias, regardless of good intentions. For example, many organisations assume refugees should be placed with diaspora populations in a receiving country. In our experience collecting preferences from refugees, a significant proportion rank diaspora connections lower than preferences around work, education, or closeness of fit with sponsor group family structures.
In addition, most matching programmes run by NGOs or civil servants boil down to a few people looking at dense spreadsheets and often making quick decisions. Bias is inherent because the inability to process and compare large volumes of data means relying on assumptions or consciously focusing on a few data points given personal experiences with previous populations or pre-determined protocols. Algorithms are a tool to alleviate these challenges.
Instances where algorithmic matching could improve resettlement experiences
We argue that in some circumstances, algorithmic matching could provide a closer fit between destinations and refugees’ attributes, goals, and preferences.
The EU’s voluntary solidarity mechanism is designed to share protection responsibility across Europe, but it is marked by political impasses and an absence of objective criteria for identifying which refugees might fare better in different destinations. Recent policy literature calls attention to the role that data and algorithms might play in responsibility-sharing.
Canada’s various refugee resettlement streams are often held up as an unmitigated success in terms of compatibility between welcoming societies and positive integration outcomes for refugees. But a significant number of newly-arrived refugees leave their places of arrival within the first year – typically for better jobs, to be close to family, or better opportunities for children. On a much smaller scale, some experience relationship breakdowns with sponsors, often because of mismatched expectations. The same trends exist with refugees in the United States.
Secondary migration and sponsorship breakdown are perennial challenges and often result in service gaps – for example, when transferring social welfare benefits between sub-national jurisdictions – and a misallocation of scarce resources. Using objective criteria to match refugees with destinations that better fit professional and social characteristics means not only a better allocation of resources but also a more immediate start on integration journeys.
Furthermore, more refined matching can help foster direct and meaningful relationships between receiving communities and refugee newcomers, and thus help build positive public opinion around humanitarian immigration programmes. Algorithmic matching offers a unique and perhaps unparalleled opportunity to collect baseline data and genuinely understand relationships between social connections and long-term outcomes – assumptions which underpin research around why sponsorship positively affects integration.
Practically, algorithmic matching ensures more robust baseline data collection (including about refugees’ preferences) and outcome evaluations that go beyond relatively simple measurements like work and language, to include refugees’ satisfaction with assigned sponsors and locations. More and better data can help unravel diverse and complex social processes by which refugees navigate social life in new communities, and those results can then be fed back into matching algorithms in order to iteratively improve outcomes. This type of learning for consistent program development isn’t possible when refugees are matched by hand and records are incomplete or subjective.
Ethical algorithmic matching in practice
Our projects in North America and Europe have offered the opportunity to reflect on some overarching lessons for the ethical use of algorithms.
- Ensure you have the right expertise
Staff who conceptualise, design and code algorithms should include experts in refugee resettlement, ethics of collecting and using data from vulnerable populations, and cyber security.
Algorithm designers should work closely with partner organisations and front-line staff to ensure the accuracy and completeness of refugee administrative data, and to solicit high-quality data from community sponsors, support agencies and different levels of government. Ensuring the quality of matching inputs will lead to better and more trustworthy outputs. Cyber security experts are equally critical to protecting data and ensuring the privacy of refugees and sponsors.
Algorithm-proposed matches should be vetted by settlement organisations and either accepted or rejected by programme participants.
- Consider refugees’ preferences and agency
Algorithmic matching should consider the diversity of refugees’ preferences and offer room to exercise their agency.
An exclusive focus on economic productivity can blur humanitarian and economic or skill-based immigration programmes. Collecting data on refugees’ preferences illustrates diverse opinions on what factors should dictate placement. Throughout Europe, our programmes use interviews and preference-ranking surveys to include refugees’ agency in matching. In our most recent work with displaced Ukrainians, their preferences dictated weights assigned to matching variables. Many ranked proximity to Ukrainian diasporas and culture, higher education and opportunities for children above work experience. In turn, this left room in matching assignments for participants with higher preferences for work.
Introducing preferences-as-data can build algorithms that limit bias and minimise reliance on unverified assumptions and stereotypes. Similar to labour-market assumptions, the common and seemingly innocuous assumption that refugees prefer relocation near co-nationals or co-religionists could have ethical repercussions, especially for those fleeing discrimination due to their identity factors like ethnicity, religion, or sexual orientation and gender expression. Including refugees’ preferences in algorithms minimises these potential pitfalls.
With community sponsorship programmes, matching should also account for receiving community preferences. Sponsors provide scarce relocation resources, and maintaining their satisfaction and engagement is critical for programme success and possibly achieving wider social and political impact. In the best-case scenarios, refugees’ preferences should be given equal weight to sponsors. Admittedly, an imperfect policy environment and logistical challenges communicating with refugees in resettlement pipelines often means relying on administrative data, but even one-sided preferences bring more voices into resettlement decisions and open the door for policy change.
- Consider the ethical implications of matching
Ethics should be central to algorithm design and when considering matching implications.
Even if an algorithm is designed to provide fair and high-quality outcomes, potential ethical implications remain. Some key questions include: Does not being matched or receiving a low-quality match (which might rationally mean rejecting an assignment) preclude displaced people from resettlement or other services? When do protection or vulnerability concerns mean a quicker match is better than waiting for a higher-quality match? Should refugees opt-in to matching programmes, or is an opt-out system better if it means more will be resettled?
- Algorithm processes should be legible to outside agencies
Algorithms and the matches they produce should be legible to policy-makers and partner organisations. This means removing algorithm processes and outcomes from a black box. While the proprietary rights of algorithm designers should be protected to promote technical innovation in humanitarian realms, matching inputs and outcomes must be clear to ensure transparency.
Participants, including refugees, sponsors, implementing agencies and governments, should be made aware of the purpose and use of their data. Consent should be genuinely informed and, where possible, refugees should be allowed to refuse a match and be resettled under a traditional pathway.
- Be clear about the limitations of algorithmic matching
Any organisation or research project advocating for algorithmic matching should communicate its limitations and manage expectations.
Algorithms are tools to optimise resource allocation, but their scope is constrained by the availability of such resources. It is essential to communicate that matches can only be as good as the resettlement locations on offer, and that they reflect the diversity of sponsors and refugees. While it’s rarely possible to meet all preferences, algorithms can incorporate broad swaths of data to make the best possible matches given real-world constraints – but real-world constraints are always present.
Conclusion
Despite divided discourses around tech in migration policy, ethically-informed algorithmic solutions for refugee resettlement are something of a middle path. Demonstrating this path requires describing an algorithm’s role and purpose. They can be likened to communication platforms where refugees, hosts, and available resources can be given voice-as-data, allowing those most affected by resettlement to influence outcomes. They contribute to decision-making structures that systematically integrate ethical rules to minimise bias and ensure fairness. They also serve as banks of knowledge that can store and sort valuable data for policy-makers and researchers to develop more effective refugee resettlement programmes. Algorithms are neither a silver bullet nor a scapegoat; they are one tool among many for fair and effective policymaking.
Ahmed Ezzeldin Mohamed
Assistant Professor of Political Science, Institute for Advanced Study in Toulouse School of Economics and Algorithm Engineer and Data Analyst, Pairity
amohamed@pairity.ca linkedin.com/in/ahmed-ezzeldin-mohamed-4b6a07a2/
Craig Damian Smith
Co-Founder and Executive Director, Pairity and Research Affiliate, Centre for Refugee Studies, York University
csmith@pairity.ca linkedin.com/in/craigdamiansmith/