- April 2024
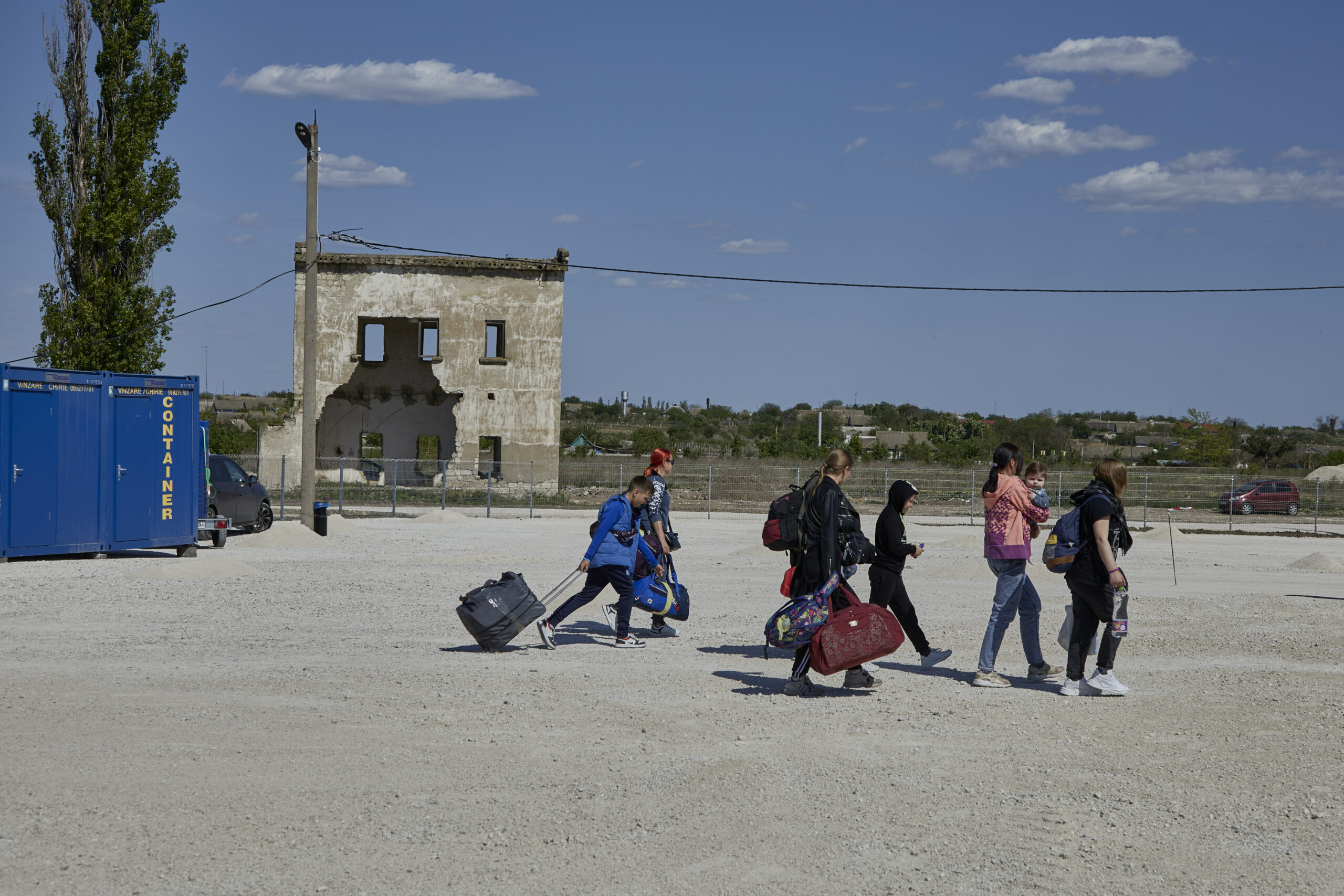
Predictive analytics to forecast future migration and displacement are receiving increasing attention, despite their limited practical utility to date. This is because they serve a number of political functions, including strengthening policy coherence and creating an impression of control.
The desire to anticipate and prepare for future developments is ubiquitous in politics. That is especially true for German and European refugee and migration policy. The recent increases in refugee arrivals via the Balkan route and the Mediterranean, and above all from the war in Ukraine, have boosted the wish not to be surprised by future migration movements. The broad interest in predictive approaches – with a strong focus on irregular border crossings and forced displacement – is reflected in a dynamic research landscape and a proliferation of competing approaches.
Quantitative forecasting tools promise better orientation and greater planning security, with instruments based on machine learning and agent-based modelling generating particularly high expectations in terms of precision and reliability. However, to date their practical utility falls short of the hopes placed in them. Considering the apparent gap between what is expected of predictive analytics and what it has to date delivered in the field of migration forecasting, why do efforts to develop related tools still attract political interest and financial resources?
The agencies involved in migration forecasting and tools used
Broadly speaking, there are three fields of application for predictions of forced displacement and irregular migration:
- boosting national reception capacity when rising numbers of refugees are expected;
- adapting border security and management to meet predicted challenges; and
- anticipatory planning of humanitarian aid (and increasingly also development cooperation) in the context of crisis-driven migration.
In all three areas, the efficient use of scarce resources is a central challenge.
A growing number of actors are involved in quantitative modelling for migration forecasting. In Europe, efforts to forecast migration tend to be concerned with refugee reception and border security and focus on those moving towards the European Union and its member States. The central actors are the European Border and Coast Guard Agency (Frontex) and the European Union Agency for Asylum (EUAA), both of which are in the process of developing machine-learning models to forecast new arrivals in EU member states in line with their respective mandates – Frontex focusing on irregular border crossings, EUAA on the number of asylum claims.
For the past few years, the EU Commission has also been investigating the potential for an EU-wide migration forecasting instrument. Apart from funding various related research consortia, it also commissioned a feasibility study on an AI-based tool to forecast the direction and intensity of irregular migration into and within the EU with a time horizon of one to three months. The results of this study have yet to be translated into practical steps; however, an instrument limited to predicting irregular migration on a single route is to be trialled in a pilot project.
The fact that none of these various tools under development is yet ready for application points to the existence of challenges that resist even the methods of machine learning. The ambition of developing a comprehensive forecasting and early warning system for irregular migration into the EU encounters technical limits in two respects. First, even the most advanced AI-based instruments currently available cannot yet adequately grasp the complex interaction of the numerous factors that influence migration decisions (especially when the respective instrument is required to be universally applicable to all countries and all migration routes to Europe). Secondly, the reliability of any forecast is limited by the inherent uncertainty of migration processes. Many of the most relevant recent migration movements towards Europe were caused by disruptive events that influenced forced displacement and migration in unpredictable ways.
Parallel to these efforts at the European level, various international organisations are developing forecasting tools for improved humanitarian and development planning, the third field of application. UNHCR’s Project Jetson, launched in 2017 to forecast displacements in Somalia, is considered the first machine-learning-based application for forecasting internal and cross-border displacement. A second initiative was launched after the Covid-related border closures of 2020 interrupted migration between Venezuela and Brazil. In order to generate viable predictions of the number of Venezuelan arrivals in Brazil after the border was reopened, and the scale of humanitarian needs this would involve, UNHCR collaborated with UN Global Pulse to create a machine-learning-based forecasting instrument and an interactive simulation tool on housing and other needs under different scenarios.
In the NGO sector, the Danish Refugee Council (DRC) and Save the Children are at the forefront of efforts to harness the potential of predictive analytics for improving aid delivery. The DRC’s Foresight Model is an AI-based tool designed to predict conflict-related internal and cross-border displacement, currently in twenty-six countries with a time horizon of one to three years. The DRC’s Anticipatory Humanitarian Action for Displacement (AHEAD) model forecasts internal displacement in Burkina Faso, Mali, Niger, South Sudan and Somalia, producing regular reports to support the operational work of humanitarian actors. Save the Children developed a machine-learning-based instrument to predict the scope and duration of displacement that has been continuously improved since its introduction in 2018. Central lessons from this process include the realisation that localised, context-specific models are more useful than a generalised global model and that certain missing data can be interpolated using agent-based modelling.
Overall, the practical use of migration forecasting in humanitarian settings seems to be more advanced than efforts to predict irregular arrivals in the EU. One reason may be that the prediction objectives are spatially and temporally more limited because of the concrete operational needs, and the learning curve more tangible than in the more Eurocentric approaches. Additionally, refugee movements caused by sudden events like natural disasters or outbreaks of armed conflict tend to be easier to model using machine learning than migration movements that are influenced by a much larger number of factors. Nevertheless, developing context-specific prediction instruments is time-consuming, requiring about one year according to the UNHCR Innovation Unit. Tools forecasting displacement may therefore be especially suitable for the longer-term observation of fragile contexts, e.g. in the context of development cooperation.
Even if the practical benefit of quantitative migration predictions varies strongly from one policy field to another, similar obstacles to feeding them into political decision-making processes exist in all areas. Despite the strong need for more forward-looking planning at all levels, national administrations lack the resources and structures required to fully exploit the findings of quantitative prediction instruments. One reason for this is a shortage of personnel and time in the relevant ministries. Another reason is that the inevitable uncertainty of predictions diminishes their value for the political process, which is best suited to dealing with clear and simple facts. And finally, there is a lack of established processes to feed forecasts into political decision-making.
Political functions of migration forecasting
So, what are the uses of quantitative migration predictions in the political process? Apart from hopes of improving inter-departmental cooperation, these include individual ministries gaining a competitive edge through the additional knowledge gained from predictions, legitimation of decisions already made, and the use of forecasts to advance political interests and to acquire funding.
Improving governmental coherence and cooperation
Decision-makers in Germany and at the European level report that the exchange of data on forced displacement and migration between different actors remains relatively unstructured and unsystematic. The patchiness of the information on forced displacement and migration hinders joint decision-making, especially in crisis situations where there is little time for coordination.
AI-based migration forecasting could contribute to a system for preparing a shared situational picture, which clearly assigns responsibilities and is accepted as a basis for decision-making by all relevant actors, and it could mitigate the time pressure that is inherent to crises by enabling discussion and consensus-building early on. The same applies at the European level, where structures for joining up the diverse migration data from different countries are notably lacking. Reliable migration forecasting could expedite coordination between the countries of first arrival, the Commission and EU agencies in the event of a rapid increase in numbers along individual migration routes.
Knowledge as a competitive advantage
Alongside the shared goal of improving predictions, there is also competition for influence over asylum and migration decisions. The fact that additional knowledge can represent a significant competitive advantage can lead to non-cooperation between different actors pursuing migration forecasting. Frontex and EUAA, for instance, both aim to leverage their predictive abilities for their own benefit: While Frontex primarily collects information about trends in irregular migration and the EUAA focuses on building reception capacity for asylum-seekers, both use their respective forecasts to garner support for institutional expansion. Ultimately, work on developing quantitative migration predictions often serves the organisation’s own political goals. This kind of ‘silo mentality’ also creates obstacles to the development of a quantitative prediction tool by the EU Commission.
Political communication and legitimisation of political choices
In the field of migration and asylum, calls for more evidence-based politics and more investment in data gathering and analysis are ubiquitous. The argument is that presenting decision-makers with evidence-based options would help objectify the frequently emotionally charged and highly polarised debates, and thus help to counteract populist rhetoric. At the same time, figures and statistics fulfil important communicative and legitimising functions in politics. Instead of serving an impartial exploration of different policy options, their primary purpose is often to legitimise or substantiate decisions that have already been taken. Quantitative predictions have yet another function: investments in migration forecasting can create an impression of control in a policy area characterised by uncertainty and periodic shocks and therefore signal efforts to realise forward planning. If forecasting efforts are primarily motivated by these considerations, they are likely to amount to a selective and largely self-serving collection and analysis of data.
Political lobbying and funding acquisition
In the humanitarian sector, quantitative predictions of forced displacement fulfil the important additional function of generating political attention for emerging crises and mobilising the required funding. The Danish Refugee Council and Save the Children explicitly name funding as one of their motives for developing forecasting tools, while at the same time trying to avoid perpetuating the narrative of a growing threat to affluent states through large-scale refugee movements from the so-called Global South. Migration forecasting can also assist state actors in allocating funding and resources. Funding invested before a crisis has broken out is a great deal more efficient than emergency relief after the event. This has long been recognised in humanitarian aid and would warrant greater use of forecast-based financing instruments. Greater efficacy of employed funds is an important argument in the competition for public resources.
Finally, humanitarian aid practitioners also emphasise that reliable predictions of refugee movements could encourage donors to allocate more unrestricted and ‘soft earmarked’ aid funding, opening up greater manoeuvring space for aid organisations to respond to needs on the ground.
Conclusion
The political functions of quantitative migration prediction outlined here underline the diverse, sometimes contradictory, motives that drive interest in forecasting migration and displacement and thus supplement their practical applications in adapting reception capacities, adjusting border protection and improving the planning and implementation of humanitarian aid and development-oriented projects. While a focus on gaining knowledge steers actors towards coordination and exploitation of synergies, the wish for competitive advantage and the legitimisation of existing policies mitigate towards unilateralism.
A discussion of the potential and pitfalls of novel forecasting tools would be incomplete without engaging with the potential negative effects of these new technical developments on migrants and refugees. While forecasting tools based on machine learning typically gather group-based rather than individual data, this still entails considerable human rights risks. In the context of the overheated and polarised debate about forced displacement and migration, quantitative predictions are inherently political and there is a risk they could be used to conjure threat scenarios and to stoke fears. This may lead to the closure of border crossings to asylum seekers, or an increase of racist attacks against particular nationalities or ethnicities. In addition, there is a lack of clarity about the rights of displaced persons and refugees to prevent their data being used in training sets for modelling migration or to receive redress if they suffer unintentional harm through the use of prediction models or if their data is misused for other purposes.
The effects of migration forecasting on forcibly displaced people can be positive (e.g. by improving humanitarian aid planning) or negative (e.g. by redirecting humanitarian funds to border security). This means that even if predictive tools gradually improve, forced displacement and migration will remain areas where difficult political decisions have to be made and defended. Where quantitative migration predictions are used in practice, the risks to those directly affected must be considered and addressed. On the one hand, it must be ensured that predictions are not used as a political tool or used to present migration as primarily a security risk. On the other, data protection must cover group-based data as well as personal data. In the area of humanitarian aid and development cooperation, the principles of the responsible data movement should be developed and adapted to keep pace with the technological progress of the predictive models.
Steffen Angenendt
Partner, Migration Experts Groups
angenendt@migrationexperts.ch
Anne Koch
Associate, Stiftung Wissenschaft und Politik (German Institute for International and Security Affairs)
anne.koch@swp-berlin.org